Materials
Informatics
Project Type:
Date:
Project Duration:
Status:
Summary:
2022
11 Months
A tool for scientists and researchers to collaborate on the creation of new materials and experimentation data.
AI
B2B
Done
Cybersecurity
Project Background
Materials Informatics (MI)
Materials informatics is a field that combines materials science, computer science, and data science to accelerate the discovery and development of new materials. It uses computational methods and data-driven approaches to analyze, predict, and optimize the properties and performance of materials.
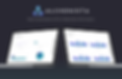
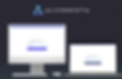
Problem
Given the proprietary nature of experimental and research data, one of the biggest challenges of the chemical industry is data collaboration between buyers and suppliers. Due to this lack of collaboration, the current time for experimentation, testing, and developing new materials can be up to 5 years, hindering innovation and market responsiveness in the industry.
One of the biggest materials manufacturers in Japan recognizes the need for a cutting-edge materials informatics tool with AI and encryption capabilities to help solve this problem.
Solution
The solution brought to the client was to create a secure and collaborative platform that integrates AI-driven materials informatics to optimize experimentation, predict outcomes, and significantly reduce the time required to develop new materials.
Key features
Alchemista provides a collaborative workspace where buyers and suppliers can share and combine experiement data, and test results securely for machine learning pruposes. The infrastructure was designed with advanced encryption protocols to safeguard experimental and proprietary data, ensuring compliance with industry standards.
As a main feature, Alchemista allows for machine learning on joined experimental data to optimize experiment parameters and predict outcomes; this particularly enhances decision-making and accelerates the innovation cycle.
Finally an intuitive user interface that facilitates seamless collaboration, data input, and retrieval between different users (companies).
My Role
The Alchemista team includes, 1 project manager, 1 AI engineer, 2 full-stack engineers, 1 encryption engineer, 1 materials informatics researcher, and myself as the designer.
As the lead designer for Alchemista, I am in charge of creating the UI based on client specifications and industry research. At the same time I have to be aligned witht the engineering team, PM and other supporting members to ensure that implementation can be matched with our homomorphic encryption engine, and data structure.
What I Did
Vision crafting
Collaborated with the project manager and the engineering team to conceptualize the vision for the MI platform keeping in mind the customer requirements for data security.
Validation discussions
Held multiple discussions with the engineering team to validate design ideas and technical feasability.
Design
Based on all the findings from previous discussions, I designed the user flows, wireframes, low fidelity and high fidelity mock ups and prototypes.
Handoff
Delivered my designs to the developers to proceed with the front-end implementation.
Client discussions
Presented multiple solutions to the customer to get their feedback and align as much as possible with their vision of the MI platform.
Design Outcome
Product architecture & User flow
It was conceived since the beginning that Alchemista would be a platform with 2 distinct users: Buyers and Suppliers. For the initial PoC, the product would have 1 screen to manage projects, 1 flow for data combination, 1 flow for model training, 1 for results optimization, and 1 las for AI prediction.

Projects
The project UI screen serves as a centralized hub where users can seamlessly access pertinent information about ongoing projects, participants involved, and a status bar indicating the project's progress. This interface provides an intuitive overview, enhancing user experience and facilitating efficient project management.
For the buyer user initiating a new project, the process begins with project creation. The user inputs essential project details, such as project name, data format, and model for trainig. Subsequently, the buyer uploads relevant experimental and proprietary data, ensuring that the robust encryption protocols guarantee the security of sensitive information. As the data upload concludes, the buyer sends an invitation to the supplier user, initiating a collaborative project.
On the supplier user side, upon receiving the invitation to join a new project, the project screen mirrors that of the buyer. The supplier proceeds to verify the data format and upload their data.
Once the supplier's data is successfully uploaded, the stage is set for the initiation of AI model training.
Model Training
From the buyer user side, the UI screen seamlessly verifies the successful integration of both datasets, and a glimpse of the dataset's general statistics. Due to advanced security measures and encryption protocols, the raw data remains confidential, ensuring the utmost protection of proprietary information. The user can navigate through essential statistics, gaining insights into the collaborative dataset's composition without compromising data integrity.
The next step is the initiation of the model training phase. With a target material property in mind, the buyer makes specifies the duration of the training, selecting the model type, and applying an appropriate scaler.
Once the model is created, the buyer can access a comprehensive training output. The UI screen showcases crucial information, including feature impact, contribution insights, and a YY plot, empowering the user with a deeper understanding of the model's dynamics and accuracy.
Simultaneously, the supplier user receives a notification upon the completion of the training process. Access is granted to visualize the same model and explore detailed statistics without compromising the security of the collaborative dataset.
This dual-access approach ensures a transparent and inclusive workflow, fostering collaboration and mutual benefit as both the buyer and supplier users actively contribute to the advancement of material innovation in a secure and efficient environment.
Optimization
On the supplier side, the user begins by selecting a previously trained model, ensuring continuity in the collaborative workflow. Subsequently, the supplier can modify feature settings, such as temperature or other properties, introducing a dynamic element to the optimization process.
A key feature of this interface is the ability to set value ranges for the selected features and establish a clear goal for the target material property, accompanied by the assignment of weight to each parameter's contribution. This nuanced customization allows the supplier to fine-tune the optimization process and align it precisely with the buyer's requirements.
The output of this optimization endeavor is presented in a clear and actionable format—a table of optimized values for the specified target. This table highlights the top values for the selected features, providing a roadmap for achieving the desired experimental outcome. By showcasing the most influential parameters, the supplier gains valuable insights into the optimal conditions necessary to meet the buyer's specifications.
This optimization process is instrumental in minimizing the iterations required for experiments, significantly reducing the materials manufacturing timeline.
Prediction
On the supplier side, the user initiates the process by uploading their specific dataset, defining the parameters for which they seek predictions. Unlike the collaborative optimization process with the buyer, the supplier's prediction process relies solely on their dataset.
A key feature of this interface is the flexibility to choose from multiple previously trained models, each reflecting a unique set of conditions and outcomes. The supplier can strategically select the most relevant models based on the similarity of experimental conditions. This approach allows the model to make predictions on optimal values and conditions for experimentation, enhancing the precision of material development.
Upon selecting the desired models, the supplier sets the minimum and maximum goals for the target material properties, establishing the framework for the prediction process. Subsequently, the user can commence the prediction, leading to an output in the form of a comprehensive table.
The information derived from this prediction process is instrumental for suppliers in streamlining their materials development process. By recognizing similarities in experimental conditions and predicted outcomes across projects, suppliers can optimize their workflow, saving time and resources.
The Results
The PoC for our collaborative materials informatics platform, Aclhemista, has yielded highly positive outcomes, with the client sharing their satisfaction and providing extensive feedback on the system's effectiveness. The successful collaboration between a single supplier and buyer has demonstrated the tangible potential of our innovative approach to materials experimentation.
The client's feedback indicates that communication and experimentation iterations have already become significantly more efficient, marking a substantial leap forward in the traditional timeline for material development.
My Reflection
Encouraged by the results of the PoC, we are now working towards a more advanced product design that will support multi-supplier projects, TEE (Trusted Execution Environment) technology for faster encryption times, and no-encryption options. This expansion aims to enhance collaboration on a broader scale, enabling multiple suppliers to contribute to and benefit from the accelerated materials innovation cycle and manage their preferred level of security.